Summary
Cyber security operations is a complex business. Each new device connected online is a target for cyber attackers to exploit, highlighting the need to find solutions to detect and defend against increasingly sophisticated attacks. This challenge is only going to become more difficult in the age of Internet of Things (IOT). The MOD collects a huge amount of network and cyber data, but the sheer volume and complexity of this data means we need increasingly automated methods to find system anomalies and flag them for action, allowing our highly skilled cyber analysts to zero in on the right things, at pace, to identify and address any issues.
The Defence Digital Innovation Team initiated an Alpha project in early 2019 to test the hypothesis that Machine Learning and Data Science can assist in making cyber security operations quicker and more effective. Building on the initial validation of the hypothesis, and working with the Service Delivery & Operations Defensive Cyber Operations Delivery Team, this progressed into a Beta phase to prove the methods against a representative MOD data set. This successfully accelerated progress in this exciting area and has led to the transition of an initial capability into Live Service.
Opportunity
MOD cyber vulnerability analysts and security teams need tools and advanced methods to support their analytical process to identify events of security interest, either device or person generated, in increasingly complex and dense network data. Surfacing events for specialist review through automated tools allows human operators to focus their efforts and use their time much more effectively.
There are many highly sophisticated cyber security products on the market which deliver new capability. The challenge to Defence is not only the cost of purchasing and licencing these products across the size of our enterprise, but also the complexity of integrating products across different suppliers that comprise an end-to-end service. Moreover, these products may be considered ‘black box’ solutions. This means that the MOD may not learn anything about how to solve the problems of the future, or how to refine the output of a jigsaw puzzle of commercial tools to improve outcomes.
Developing this capability in-house, rather than simply buying third party tools, means that we avoid the cost and complexity of implementing new tools; whilst also optimising the technical data required to input to them. We also learn how to solve future challenges as our enterprise becomes more complex and threats continue to evolve.
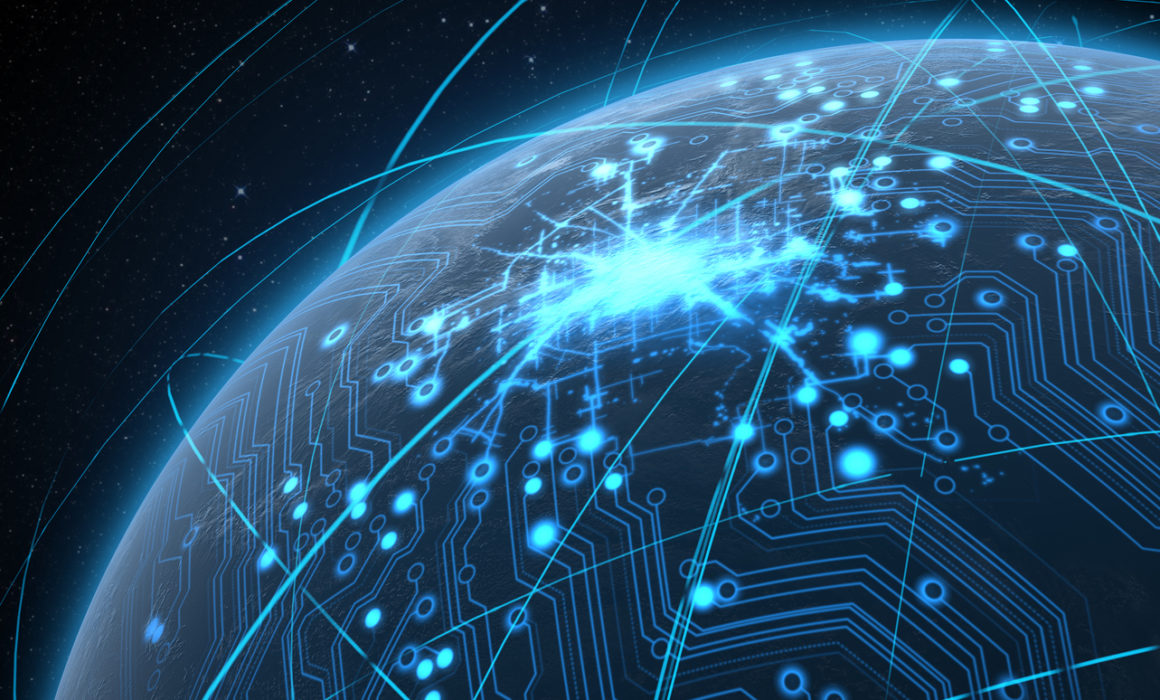
Approach
Initially, a proof of concept Alpha project using representative network data quickly proved that data science methods and machine learning techniques could be readily applied to yield results that would improve analyst efficiency and effectiveness.
Building on the proof of concept, the Beta project was conducted within the Defence Cyber Capability (DCC) Cyber Situational Awareness Fusion Architecture (CySAFA) environment using real Defence network technical data. CySAFA is a live environment which enables large amounts of data to be collated to support decision making in cyber defence.
Initially, Data Scientists on the project used exploratory data analysis to determine the content and its value captured by various data feeds - the vastness and variety of the data that is stored in CySAFA means that understanding the value of data is a challenge in itself. The subsequent fusion and correlation of various data sets then provided a rich picture of activity, upon which automated analysis techniques have been developed and applied.
For example, by implementing the "FastText" algorithm, first implemented by Facebook in 2016, to extract computer host names from free text the data scientists have been able help cyber analysts find and identify assets in multiple data sets much more rapidly, replacing what used to be a manual search process. This tool, amongst others, is now available to analysts in the live CySAFA environment.
Outcome and Impact
The beauty of this innovation project is that the solution is being iteratively delivered as a fully supported capability in to the live CySAFA environment. Having the ability to understand the data and apply existing data science techniques means that new algorithms can be developed which are specific to the Defence enterprise and tuned to user requirements.
As the innovation team activity closes out, the data science team now remains a contracted resource as part of the DCC/CySAFA capability. This local data science team will now operate as a satellite team of the Centres of Expertise (COE) for Data and Artificial Intelligence (AI), to share best practice and inform appropriate adoption of data and AI capabilities across Defence.
'The Machine Learning project has already delivered benefit to the personnel within the Cyber Security Operations Capability (CSOC). Not only has it identified data science techniques that will be of benefit in future, it has already updated the live cyber defence environment to reveal previously undiscovered knowledge contained within the data we already hold.
When Machine Learning advances are closely aligned with the operational need, the potential going forward will be huge; revealing new insights across the Defence Digital estate, improving the efficiency of our cyber defence teams and integrating our existing tooling to maximise the benefit from investments already made.' - DCC Integration and Exploitation team.
As well as being entirely aligned with Defence Chief Information Officer strategic priority for responsive cyber defence (providing robust and responsive cyber defence against an ever-evolving threat), this project supports the concept of Information Advantage enabling relative advantage over adversaries in the information environment.
1 comment
Comment by Peter Grindrod posted on
Yes, but in July 2019 there was recent call for ideas and submissions from the co-chairs, Eric Schmidt (former Alphabet CEO) and Robert Work (former deputy secretary of defense), of the US National Security Commission on Artificial Intelligence (AI). They asked a number of questions.
The Rand Corporation responded with some internal essays. The topics submitted included the following two points, which are relevant to your assertions regarding M L.
a) The need for more AI verification to inure trust. We know that opportunities for spoofing and Mal outcomes increase in probability to one, as the dimensionality of objects input increases. So theoretically this problem gets worse. This cannot be set aside.
b) Managing security threats caused by hasty implement insecure/naive systems containing advanced ML, introducing dangerous vulnerabilities that will be exploited. Again this requires work on fundamental limits of ML and AI.
There is much work to do and you should confront it, not seemigly trying step around it. I think the UK should take a lead here.
A more realistic assessment of some of they challenges is here:
https://defenceindepth.co/2020/08/11/on-mod-ai-and-data-science/